Quick Summary: In the digital landscape, machine learning stands as a pivotal subset of artificial intelligence, empowering computers to learn and improve from experience autonomously, without explicit programming. By leveraging the data, machine learning drives innovation and shapes the evolution of technology across industries. Explore the working process, applications, types, benefits and trends of this transformative field.
The concept of Machine Learning has been a buzzword in recent times and is revolutionising the digital world. It is now prevalent across industries, with organisations eager to leverage this technology and create a blooming opportunity among data scientists.
Let’s get started!
What is Machine Learning?
Machine learning is a branch of artificial intelligence where machines are trained to make decisions and act intelligently without the intervention of humans.
Arthur Samuel, the father of machine learning defines it as “The field of study that gives computers the ability to learn without being explicitly programmed.” These machines can analyse tasks, figure out the decision and use the information for a better outcome.
Are you aware that you engage with machine learning daily?
Consider this: Have you ever wondered how Google Maps can predict the traffic accurately? How does it inform you about traffic on 12th Street due to ongoing metro construction? Google Maps uses real-time data to analyse the traffic, road conditions and patterns. Machine learning extracts information from geo-located imagery using a vast collection of images to make this possible!
Machine learning automates the process of learning from data. You give the data, it breaks the number, makes predictions or even takes actions without the need for constant reprogramming. It learns from new information and improves predictions over time.
Here is an interesting stat on Machine Learning. The market size of machine learning is projected to reach US $204.30 billion in 2024 and is expected to grow to US $528.10 billion in 2030 at a CAGR of 17.15%.
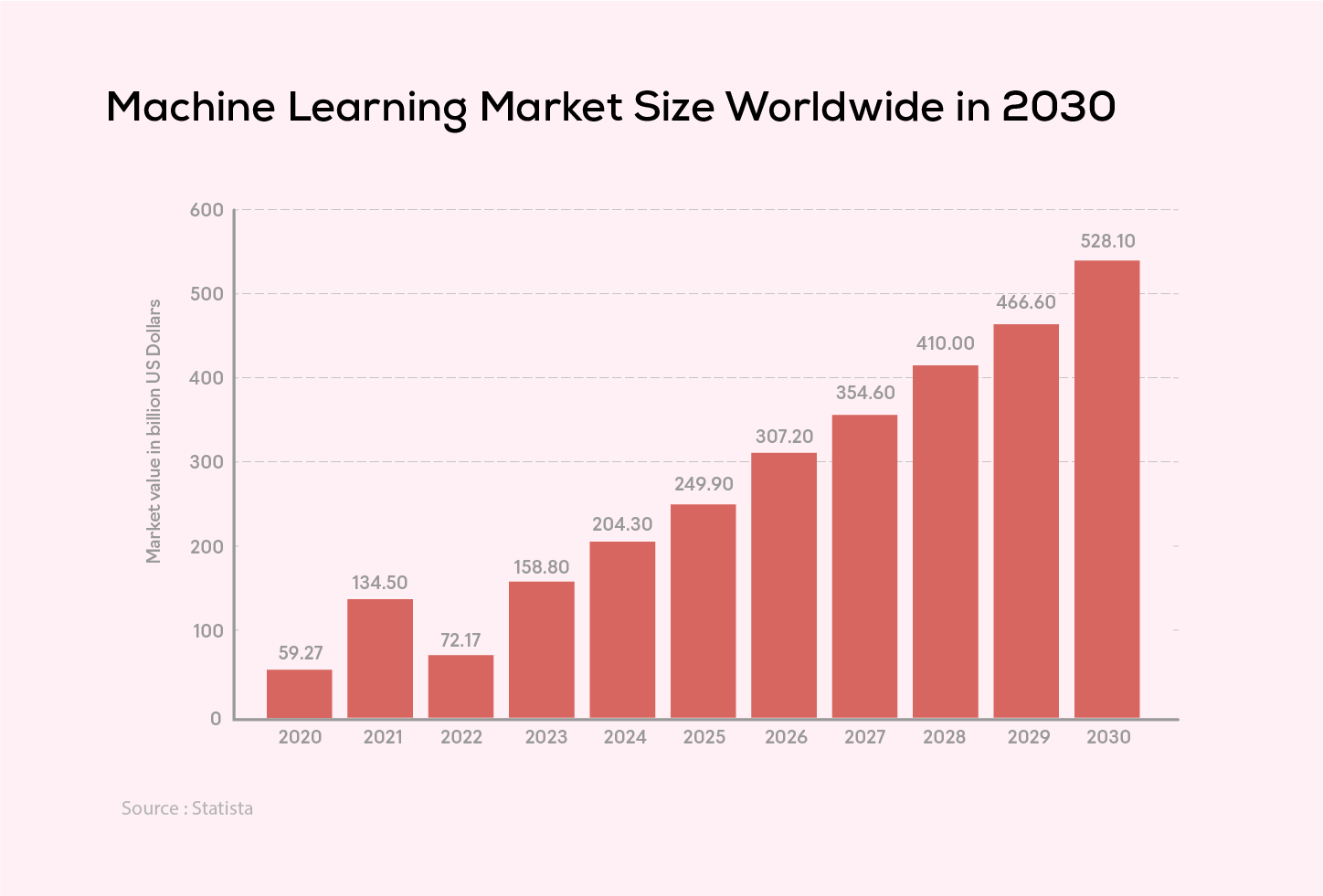
How Does Machine Learning Work?
Machine learning, a subset of AI, enables machines to improve their performance over time by learning from data. The process begins with feeding training data into the system, which helps the program learn and enhance its capabilities. The quality of this training data significantly influences the effectiveness of the machine learning process.
To evaluate performance, new input data is tested against the machine’s predictions. The program then compares these predictions with actual results to refine its accuracy. If there are discrepancies, the algorithm is adjusted and retrained until it achieves the desired outcomes. This iterative process allows the program to continuously learn and improve its predictions.
Types of Machine Learning
Machine learning can be broadly classified into three types based on the nature of the learning system and the available data: supervised learning, unsupervised learning and Semi-supervised learning. Let's explore each in detail:
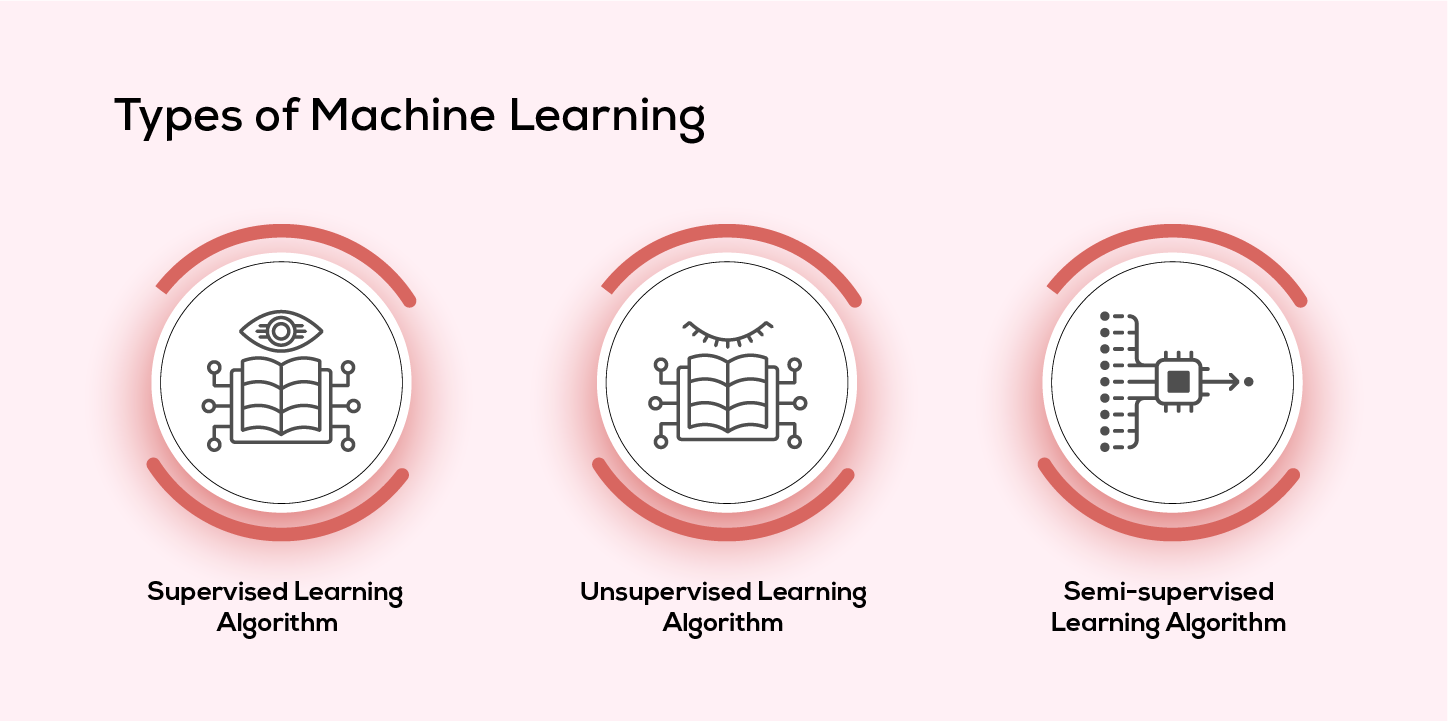
1. Supervised Learning
In supervised learning, labelled data is used to train the program to recognise patterns and make predictions independently. The labelled dataset clearly defines the information the program should focus on and the type of result it should produce. This process involves mapping input to output data, allowing the algorithm to learn the relationship between them and improve prediction accuray.
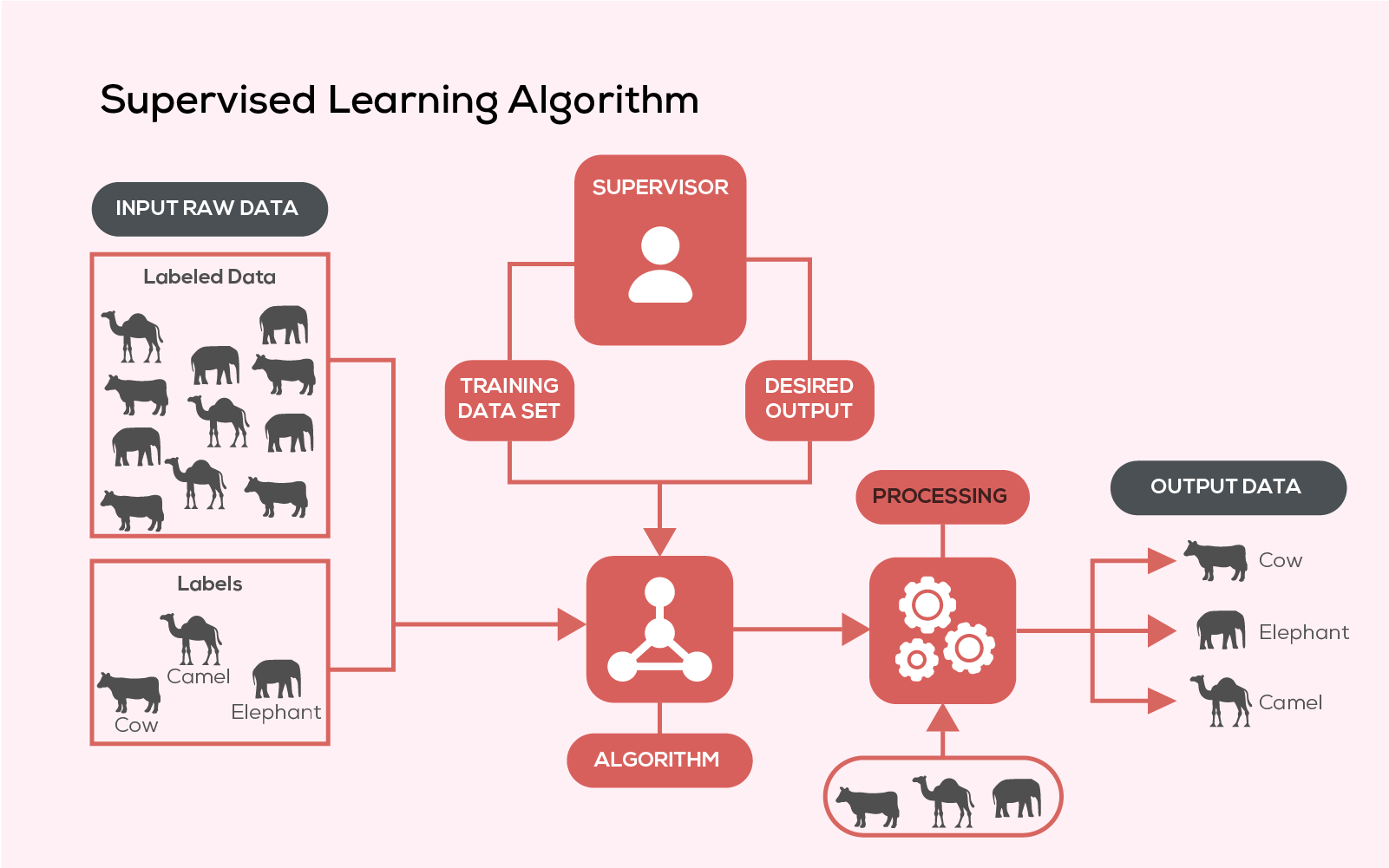
Here the model is trained on the labelled dataset each having input and output parameters. The input data serve as clues or details whereas the output data represent the final targets.
Here are some of the top algorithms used in supervised learning:
- Random Forest
- Logistic Regression
- Naive Bayes
- Decision Trees
2. Unsupervised Learning
Unsupervised machine learning allows the model to learn from data without human intervention. It uses unlabelled data to find patterns and connections independently, without explicit instructions. The goal of unsupervised learning is to discover hidden patterns in the data. Unsupervised learning works by interpreting the hidden patterns and groups, then using those patterns to categorize new data. These algorithms find and extract useful information that results in better decision-making. These algorithms learn from the data and use the knowledge to create categories to group data.
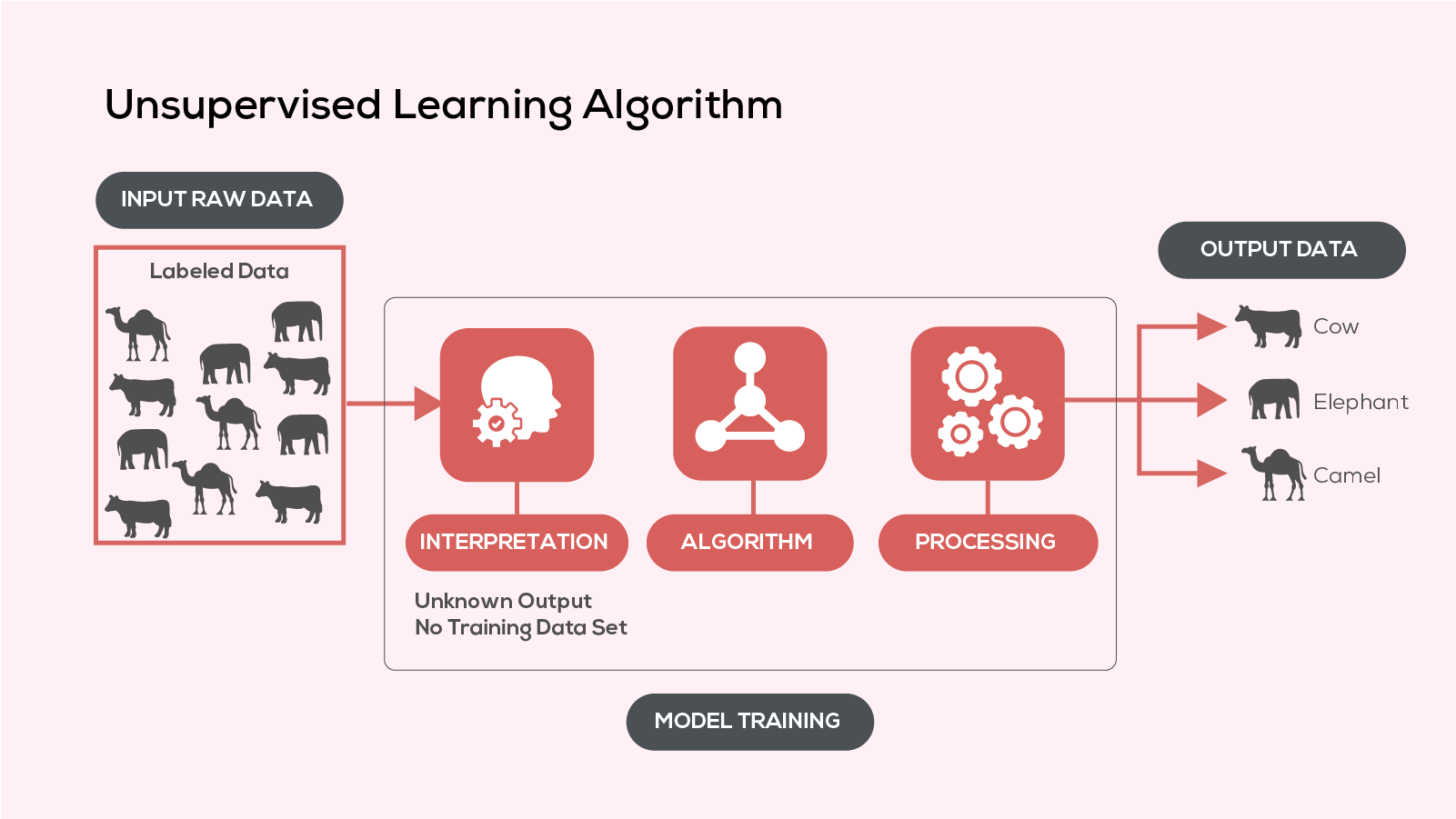
The common use cases of unsupervised learning are:
- Fraud Detection
- Anomaly Detection
- Genetic Research
- Customer Segmentation
3. Semi-supervised Learning
Semi-supervised learning blends elements of both supervised and unsupervised learning. It leverages a small amount of labeled data to guide the learning process while using a larger set of unlabeled data to improve the model's effectiveness. This approach allows the program to analyze data, discover hidden patterns, and learn more efficiently.
The goal of semi-supervised learning is to combine the strengths of both methods, uncovering hidden patterns and generating valuable insights across various fields.
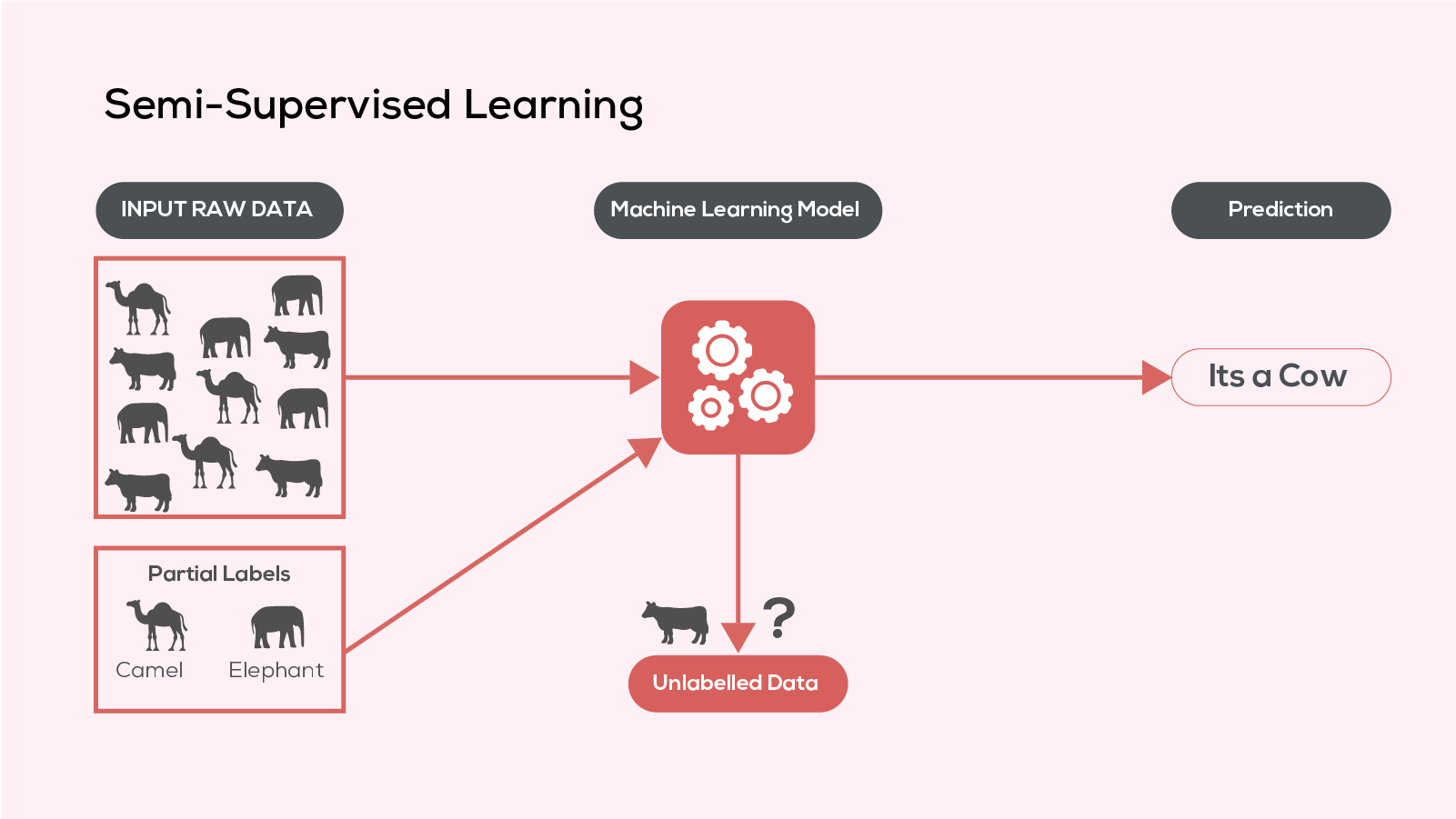
The real-life use cases include:
- Speech Analysis
- Image and Text Classification
- Internet Content Classification
Real-Life Use Cases of Machine Learning
Machine Learning is revolutionising the world around us and is changing the way we interact with technology. Machine Learning is a powerful tool that businesses use to improve decision-making, boost productivity and even detect diseases. Here are some of the real-world use cases of machine learning:
1. Image Recognition
With the rise of smartphones, everyone has become a photographer, capturing and sharing moments like never before. Social media platforms, apps, and websites are flooded with images, with 71.9% of content on Instagram being photo posts.
Machine learning powers image recognition, enabling computers to identify objects within images. Organizations use algorithms such as neural networks and deep learning models to analyze data from photos across various fields, including healthcare, e-commerce, transportation, and marketing.
According to a survey by MarketsandMarkets, the image recognition market is projected to grow from USD 46.7 billion in 2024 to USD 98.6 billion by 2029 at a CAGR of 16.1% during the forecast period.
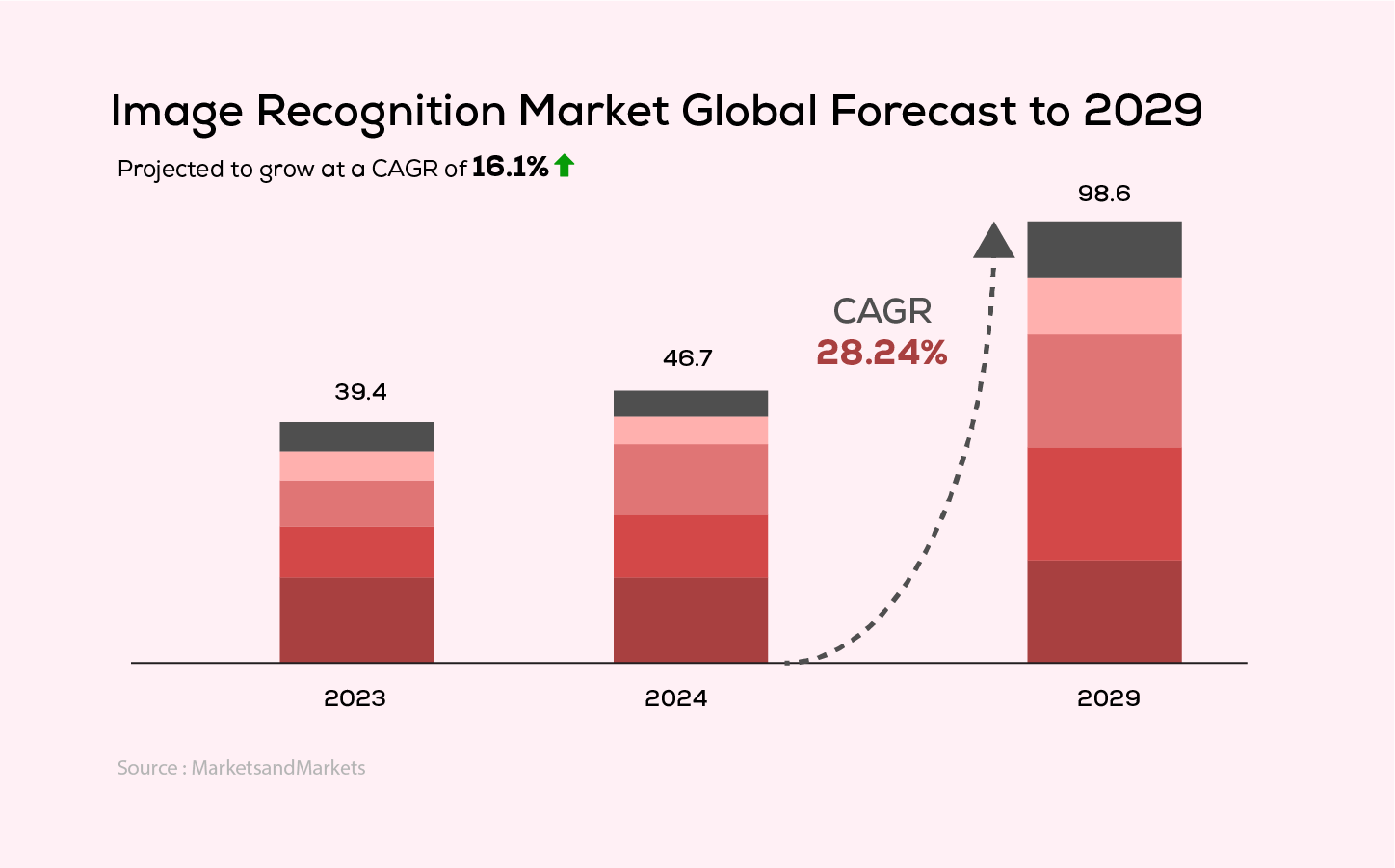
2. Speech Recognition
Have you ever wondered how virtual assistance like Siri, Alexa, or Google Assistant understand and respond to your commands? The answer lies in speech recognition. a branch of artificial intelligence that enables machines to comprehend and act on human speech. This technology allows devices to convert spoken words into text, execute tasks, and interpret commands.
Speech recognition is widely used in various fields, including customer service, voice biometrics for security, marketing, and healthcare.
Take a look at the digital assistants used by consumers.
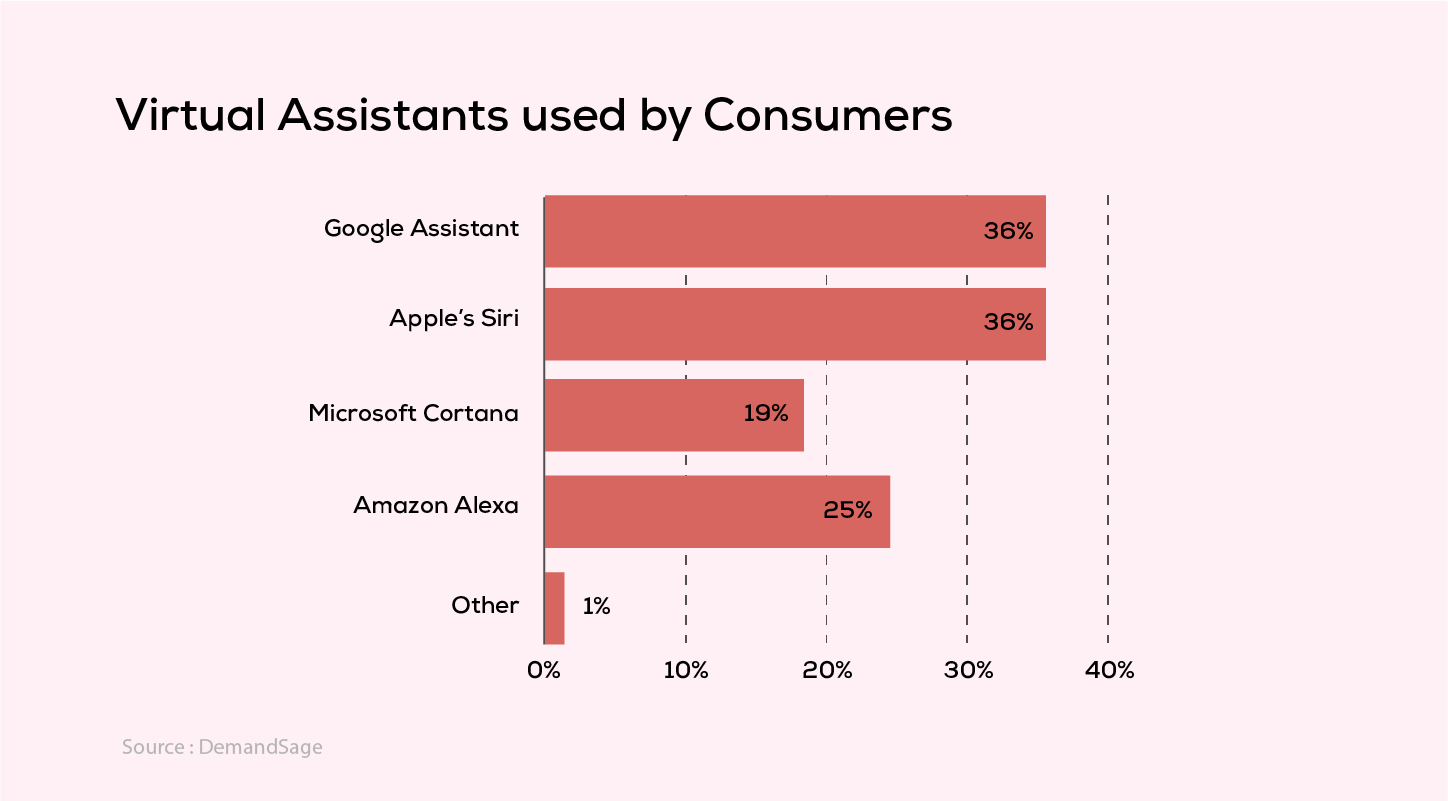
3. Self-driving Cars
Have you ever imagined a world where the car would drive you to the destination without your help and you sit back and relax? Here comes the future of transportation – Self-driving cars!
Autonomous vehicles (driverless cars) are designed particularly to function without human intervention. They rely on machine learning technology, using various sensors like LiDAR, GPS, Radar, Sonar and odometry to assess their surroundings. These sensors enable the vehicles to recognise objects like pedestrians, road signs, animals and other vehicles. Through object recognition, self-driving cars can interpret shapes and obstacles on the road, ensuring safe and efficient travel.
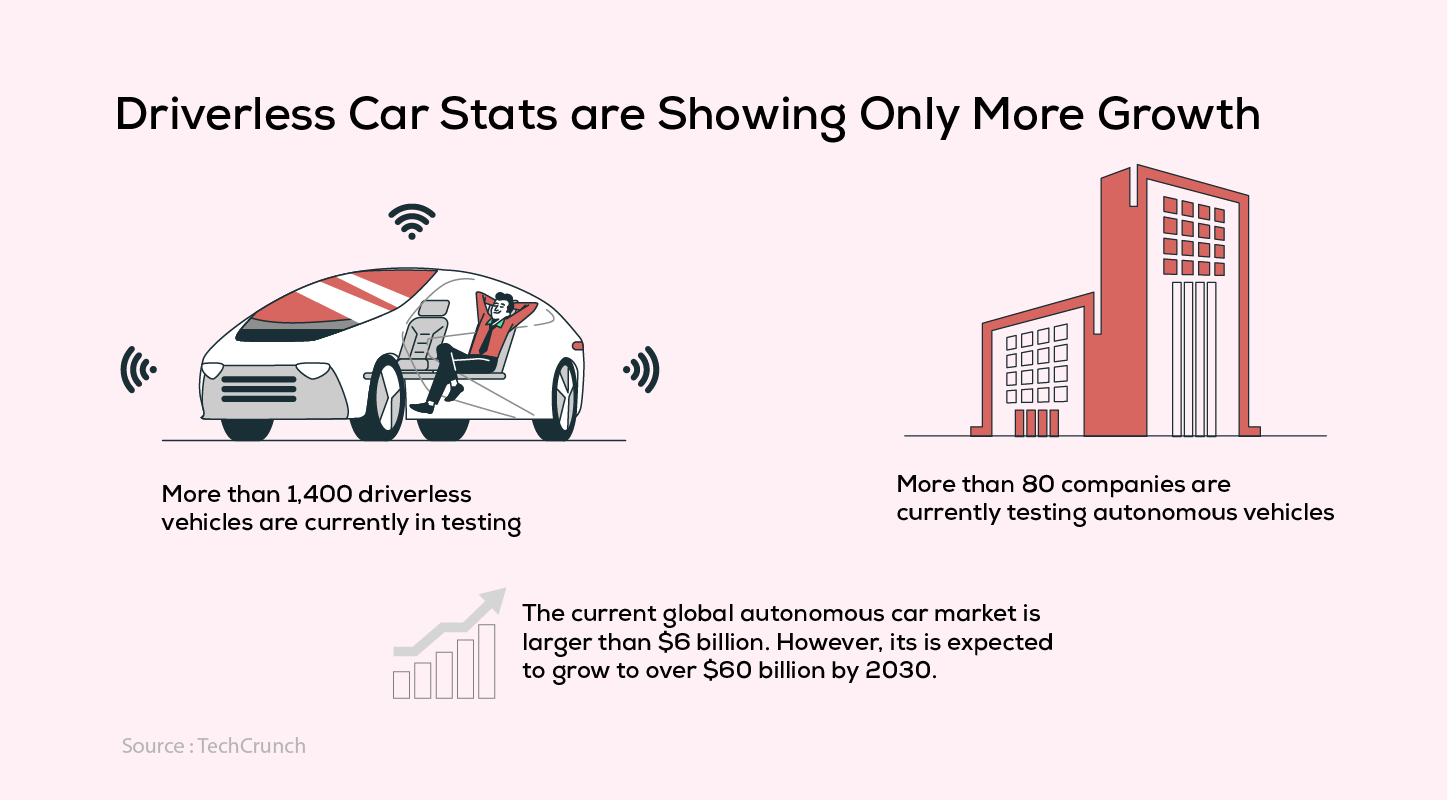
4. Sentiment Analysis
Sentiment analysis uses machine learning to determine whether a piece of text is positive, negative, or neutral. By training machine learning models with examples of emotional content, these tools can interpret how people feel based on their writing. Organizations commonly use sentiment analysis to assess online reviews, customer feedback, call center interactions, and social media posts.
Discover the transformative potential of Inspirisys' sentiment-based Predictive Analytics platform - SocioMentis. Elevate your strategies with invaluable insights, driving your success forward. Embrace data-driven decision-making to stay ahead in your industry.
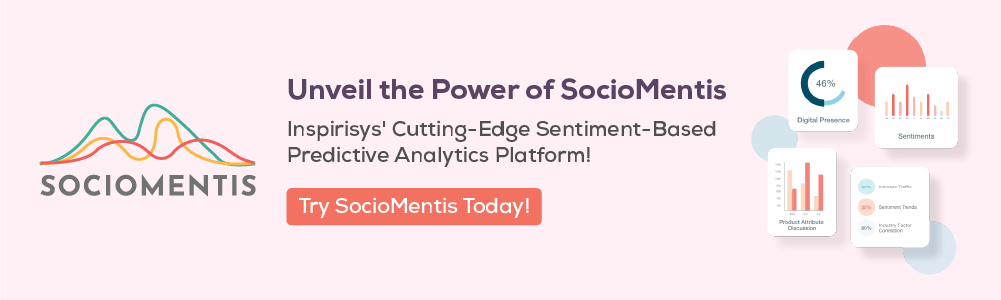
5. Social Media
Ever wondered how social media platforms seem to understand your preferences so well? Well, machine learning is the secret. These platforms learn your interests, tailor your news feed and deliver content suited to your tastes.
Platforms like Facebook and Instagram utilize machine learning algorithms to analyze data, uncover hidden patterns, and even predict what products you might be interested in. By integrating these analytical tools, social media platforms can better understand and respond to online preferences.
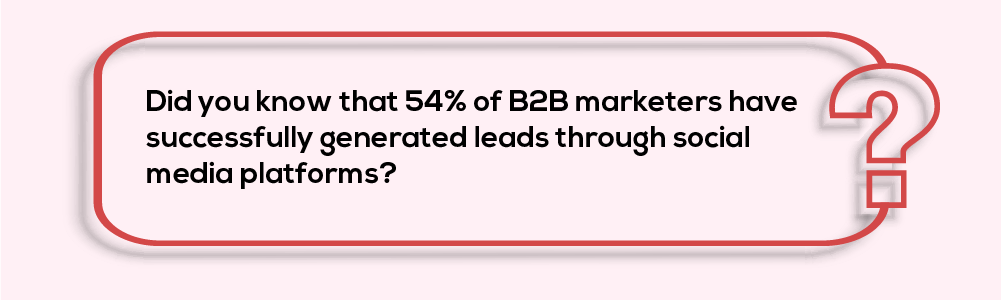
Importance of Machine Learning
In today’s world machine learning has become a vital tool across various industries. Let’s dive into the reasons why it’s so important:
1. Data-driven Insights
Machine learning helps businesses to uncover hidden patterns and insights from customer data. By analysing large datasets, organizations can identify market opportunities, make informed decisions and understand trends more effectively.
2. Automation and Efficiency
The key benefit of machine learning is automation and efficiency. It can automate both repetitive tasks and complex calculations, saving time and effort while minimizing errors. Further, it helps businesses streamline operations and reduce costs.
3. Enhanced Security
Machine Learning helps to identify and stop threats before they cause damage. It aids in constantly monitoring network traffic and user behaviour. This allows them to identify and prevent cyberattacks.
4. Healthcare Advancements
Machine learning is playing an important role in the field of healthcare industry. It is being used in hospitals to improve disease diagnosis, drug development and personalised care for patients. The predictive models help doctors make better diagnoses, while AI research helps in the discovery of new medicines.
5. Predictive Analysis
Machine Learning models use past information to make predictions in the future. Predicting future trends, helps them estimate sales, plan for demand and manage risks more effectively.
Recent Developments in Machine Learning
- Predicting Future Water Availability:
With the rising global population and increasing demand for freshwater, machine learning has become a vital tool for predicting water availability. It enhances accuracy in forecasting the water cycle by employing techniques such as artificial neural networks (ANNs), support vector machines (SVMs), and deep learning models.
Machine learning algorithms analyze various factors, including rainfall amounts, temperature, and soil type, to predict groundwater levels, precipitation, and streamflow. By integrating these factors, machine learning creates a comprehensive understanding of our water resources.
- Transforming Trauma Care:
Researchers from Osaka University are leveraging machine learning to enhance trauma care by identifying patients who are likely to benefit from a drug that stops excessive bleeding. By analyzing data from 50,000 patients, they have developed a predictive model for the medication tranexamic acid, which helps control bleeding.
Machine Learning Trends
In today’s fast-evolving technological landscape, both Artificial Intelligence (AI) and Machine Learning (ML) are pivotal. Machine Learning simplifies tasks, accelerates processes, and reduces errors, revolutionizing industries such as healthcare and finance through innovation. As we advance into a new era, these technologies continue to expand and reshape various sectors. Here are the latest trends in Machine Learning:
1. Low-Code or No-Code Development
AI and machine learning are revolutionizing industries such as agriculture, finance, marketing, and banking by enhancing efficiency. In 2024, low-code and no-code platforms are advancing, allowing users without coding experience to leverage these technologies. These budget-friendly platforms are increasingly used for tasks like employee hiring, customer sentiment analysis, and demand forecasting. As these platforms continue to evolve, they enable developers to create powerful, user-friendly machine learning applications, improving the overall user experience.
2. Metaverse
The metaverse is rapidly advancing and capturing global attention with its interactive experiences. Businesses are leveraging this virtual realm to engage customers more effectively. As the metaverse evolves, machine learning plays a crucial role in driving its development and expanding its possibilities. With ongoing technological advancements, the metaverse is increasingly becoming an integral part of our daily lives.
3. MLOps
MLOps streamlines the process of building, testing, and deploying machine learning models. By integrating best practices from machine learning, DevOps, and data management, MLOps ensures efficient transitions from model development to real-world applications. It emphasizes automation to speed up and enhance the reliability of machine learning processes.
The global MLOps market, valued at USD 1.4 billion in 2022, is projected to reach USD 37.4 billion by 2032, growing at a CAGR of 39.3% from 2023 to 2032.
Wrap Up
The world of machine learning is rising at a high speed. The field is constantly reshaping and evolving, with exciting new trends emerging over time. Machine learning is creating a world of possibilities and opening the door to exciting new developments. It’s making our lives smarter, transforming industries like healthcare and finance, and helping us solve challenging problems. This technology is changing the digital world and winning the game.
The future is here!
Frequently Asked Questions
1. What is the difference between machine learning and deep learning?
Machine Learning typically involves simpler algorithm structures, such as decision trees or linear regression. In contrast, Deep Learning utilizes complex artificial neural networks.Additionally, Machine Learning often requires manual intervention for tasks like image sorting, whereas Deep Learning can achieve these tasks with minimal human input due to its ability to automatically learn and extract features from data.
2. How is feature selection important in machine learning?
Feature selection is the process of eliminating irrelevant or redundant details from the data, which enhances the performance of machine learning models. It improves model accuracy, reduces training time, minimizes overfitting, and enhances model interpretability.
3. How does transfer learning work?
Transfer learning involves using a pre-trained model to address smaller-scale problems. It leverages a model that has already been trained on a large dataset, such as images. The model is then fine-tuned for specific tasks, allowing it to learn faster and perform better on new, related problems.
4. What are the challenges of machine learning?
The challenges of machine learning include data underfitting, poor quality of data, irrelevant features and maintenance.
5. What are the best languages for machine learning?
The challenges of machine learning include data underfitting, poor quality of data, irrelevant features and maintenance.